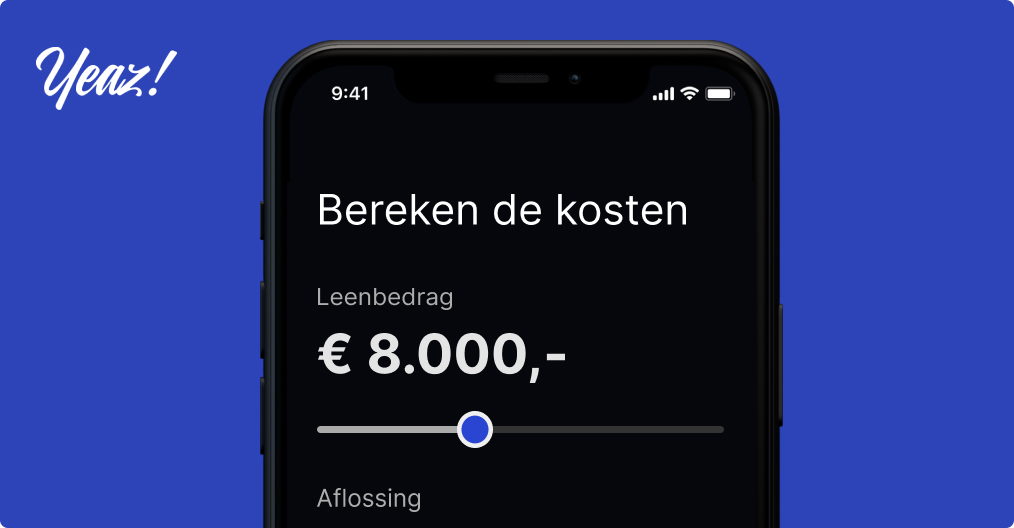
Mobicom — MonPay Loan
An easy-to-use AI-based credit scoring system to provide microloans with minimum risk by leveraging Big Data
Challenge
Our customer Mobicom is a large Mongolian-Japanese venture. The telecommunication company covers over one-third of the mobile service market. Recently, the company launched Mobifinance Non-banking financial institution. It allows end-customers to apply for instant loans, get approved, and receive finance in no time.
The loan decision is based on a large amount of data, available to the telecommunication provider: such as payment history or usage statistics.
The goal was to improve risk management and increase profits. The existing solution could not help Mobicom reach the planned results. It required a lot of time and efforts from the team
The customer was searching for a powerful credit scoring product, which is able:
- To work with Big Data
- Evaluate the impact of certain data types on the output
- Create multiple models for experimenting and choosing the market strategy
- Take the maximum of what AI and ML can offer to build high-quality predictive models.
Approach
The GiniMachine team gathered customer’s requirements and explained the system implementation step-by-step. Fast onboarding and easy use of the product was of primary importance for the Mobicom team.
These objectives can be reached due to a set of heuristic methods along with a custom implementation of the decision tree ensemble method.
Such a combination helps to apply modern ML techniques to almost any raw data set and automate technical redundant tasks like data preprocessing.
This way company’s risk officers and data analysts get more time left for creative tasks, experimenting, analyzing the resulting models. With the ready-to-use dataset, GiniMachine creates and validates a scoring model in 2-10 minutes, depending on the amount of data.
Result
Mobicom telecommunications company managed to reach its objectives and now it can grant loans in several clicks with the optimum ratio between risks and possible profits.
The customer’s choice was to deploy the credit scoring system on-premises. It took a few additional efforts related to the allocation of server capacity and balanced server performance. The GiniMachine team detected the issue at a glance, helped to fix it at the testing stage, so the solution started building scoring models and showing Gini Index more than 0.6 right after, which is a good result.
The Mobicom team notes the high performance and reliable prediction power of models. It required no special training to start working with the solution. GiniMachine uses unstructured data, big data, process missing data, and find non-obvious dependencies.
COVID-19 added certain challenges to the project, but the whole idea of fueling online lending and helping consumers stay home is important and socially valuable.
As a result, the customer benefits from fewer non-performing loans and higher acceptance rates. The tool can offer insights into lender’s data and can be used for exploratory analysis.
Openness and access to information allows others to get access they otherwise would not have had, and to influence product direction in ways they weren’t able to previously. That’s incredibly powerful.
Matthew Horan, Staff Engineer at Spotify